Integrating CFA Principles with Machine Learning in Finance
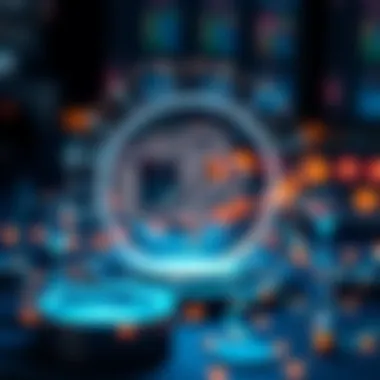
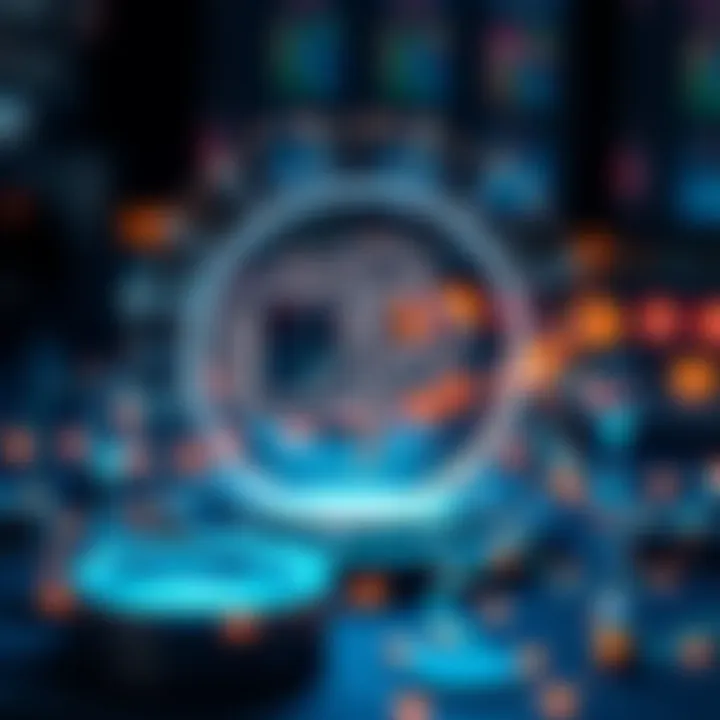
Intro
In the realm of finance, an era of transformation is underway. The collision between Chartered Financial Analyst (CFA) principles and machine learning is creating ripples that are difficult to ignore. These two robust fields, traditionally seen as separate, are now weaving tightly together, fostering new methodologies and insights that significantly enhance financial decision-making. This integration is not just a passing trend; it is reshaping investment strategies, risk management practices, and overall financial analysis.
The CFA designation is recognized globally, embodying rigorous financial analysis and investment acumen. Machine learning, on the other hand, provides powerful tools that can process vast amounts of data quickly, revealing patterns and insights that might elude human analysts. The marriage of these two elements offers a promising avenue for improving accuracy in investment forecasting, portfolio optimization, and understanding market intricacies.
This article sets out to dissect the nuances of integrating CFA principles with machine learning. We'll explore how these technologies can enhance traditional analytical methods, scrutinize the impact on investment strategies, and delve into the practical implications for risk management. By the end of this exploration, one should appreciate the significance and the multifaceted opportunities that arise from this integration, ensuring that financial analysts, individual investors, and technology enthusiasts are well-equipped in today’s dynamic landscape.
Let’s take a dive into the foundational concepts before unraveling the complex tapestry of advanced methodologies.
Preface to CFA and Machine Learning
In today’s fast-paced financial landscape, Chartered Financial Analysts (CFA) and machine learning (ML) are emerging as critical components for effective investment and analysis strategies. Combining these fields is not just a passing trend—it's a necessary evolution that holds the potential to redefine the boundaries of financial analysis.
Why This Matters
Integrating CFA principles with machine learning techniques enhances the way investors and analysts interpret data, forecast trends, and ultimately make decisions. Modern finance is inundated with vast amounts of data, and relying solely on traditional CFA methodologies may hinder one’s ability to extract actionable insights. By leveraging machine learning, practitioners can better manage complex datasets, uncover hidden patterns, and predict market movements with greater accuracy.
Key Elements to Consider
The significance of intertwining these two spheres cannot be overstated. Here are a few specific elements to reflect upon:
- Data-Driven Insights: ML algorithms can sift through piles of data more quickly than a human analyst, allowing for insights that are driven by evidence rather than intuition.
- Efficiency: Manual analysis often consumes substantial time and resources. By employing machine learning tools, analysts can focus on higher-level strategic discussions instead of being bogged down by data crunching.
- Predictive Power: Integrating CFA's understanding of financial metrics with ML’s algorithms provides a robust foundation for predictive capabilities. This means not just observing trends but anticipating them, pivoting if necessary, based on the insights gleaned from data.
Considerations for Implementation
Adopting a hybrid approach requires careful consideration. Analysts need to grasp both the fundamentals of financial theory found in CFA and the technical aspects of machine learning, which can seem daunting at first. Furthermore, questions about data quality, model interpretability, and the risk of overfitting must be navigated. Simply put, while the marriage of CFA and ML is promising, achieving a seamless integration calls for a solid understanding of both disciplines.
By exploring the subsequent sections on defining CFA and outlining the fundamentals of machine learning, readers will gain a better grasp of how each contributes to a richer analytical process. Ultimately, the goal here is to foster a deeper understanding of how marrying CFA methodologies with machine learning not only enhances financial acuity but also positions analysts on the cutting edge of industry practices.
The Convergence of CFA and Machine Learning
Exploring the convergence of Chartered Financial Analyst (CFA) principles with machine learning provides a deeper understanding of how these realms can work hand in hand to revolutionize financial analysis. The traditional CFA frameworks focus on analytical techniques and investment principles. Meanwhile, machine learning offers a new lens through which to interpret vast datasets and uncover patterns that often escape human analysts. The melding of these two domains invites a fresh approach towards investing, risk management, and portfolio optimization.
Why Combine CFA with Machine Learning?
The integration of machine learning into CFA practices isn't merely a trend; it’s a significant upgrade. Investment firms that shy away from incorporating machine learning into their analytic toolkit might soon find themselves outpaced by competitors who adopt these technologies. One of the core reasons behind this fusion lies in the ability of machine learning algorithms to analyze vast quantities of data at unprecedented speeds. The speed and accuracy of these algorithms can lead to better decision-making, especially in volatile market conditions.
Moreover, machine learning brings flexibility to the analysis. Unlike traditional methods, which often rely heavily on statistical assumptions, machine learning can adapt and improve as it is exposed to new data. This adaptability is crucial in finance, a field constantly influenced by changing economic indicators, trends, and unexpected events.
Benefits of Integration
Enhanced predictive capabilities
Enhanced predictive capabilities stand out as one of the most important advantages of merging CFA with machine learning. By utilizing algorithms that can process and learn from historical data, financial analysts can forecast future trends with higher precision.
The key characteristic here is the algorithm’s ability to assimilate inputs from various diverse sources—ranging from stock prices to macroeconomic indicators. This aspect makes enhanced predictive capabilities a beneficial choice for investors seeking an edge in their strategies. Instead of relying on gut feeling or simple linear models, analysts can leverage machine learning to uncover complex relationships within datasets that they might not have considered before.
However, it's crucial to maintain a balance between model complexity and interpretability. While sophisticated models can yield better predictions, they might be less transparent, making it harder for analysts to explain their decisions to stakeholders.
Data-driven decision making
Data-driven decision making reflects another compelling benefit of integrating CFA with machine learning. Accumulating evidence suggests that firms who base their strategies on sound data analysis tend to outperform their peers. This method removes a significant amount of guesswork from the investment process.
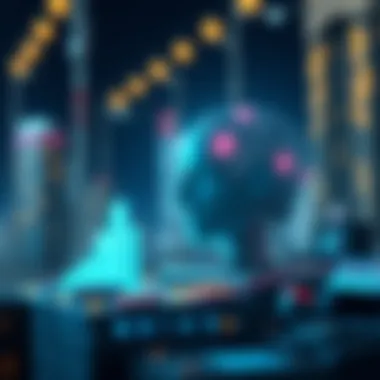
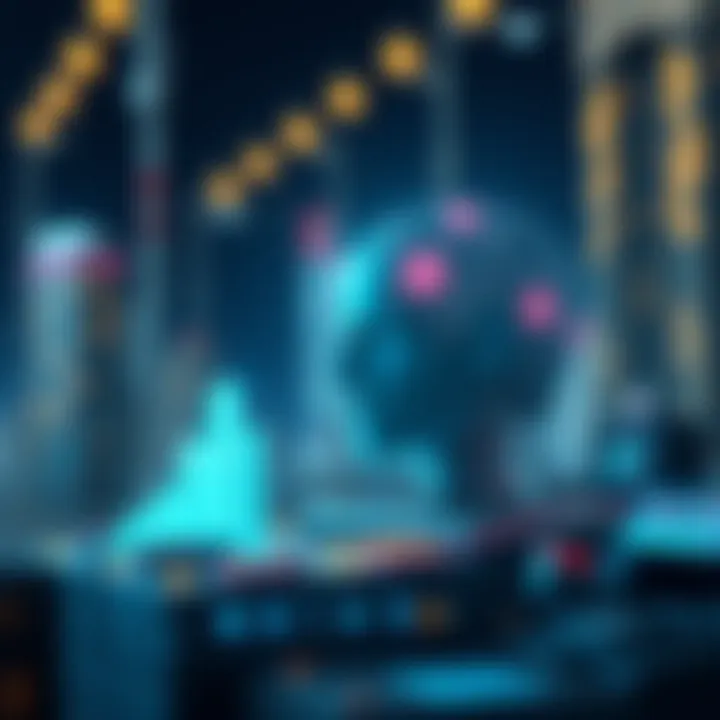
This approach prioritizes empirical data over anecdotal insight, which is a powerful shift in mindset. The unique feature of data-driven decision making lies in its ability to provide objective insights. As a result, financial analysts can make informed decisions backed by numbers, increasing their chances for success.
Yet, it’s worth noting that heavy reliance on data can bring its own set of challenges. Analysts must ensure the quality and accuracy of the data they’re using. Misleading or incorrect data can skew conclusions in the blink of an eye.
Efficiency in analysis
Lastly, efficiency in analysis emerges as a crucial advantage in the context of integrating machine learning with CFA. Traditional analysis methods often require substantial time and human resources. Machine learning can streamline these processes significantly, allowing analysts to focus on strategic decision-making rather than data crunching.
The ability to automate routine tasks means financial professionals can devote time to interpreting results and strategizing. Efficiency appears particularly beneficial when rapid responses are required in today’s fast-paced financial environment. However, while the efficiency garnered from this approach is notable, it must not come at the expense of thoroughness. Analysts should remain vigilant, regardless of how fast the process becomes.
Collectively, the integration of CFA with machine learning embodies the evolution of financial analysis. By understanding and navigating the convergence of these two fields, CFA professionals can position themselves at the forefront of financial innovation.
Machine Learning Methodologies Relevant to CFA
Integrating machine learning methodologies into the realm of Chartered Financial Analyst (CFA) practices can significantly enhance how financial data is interpreted. Among the multifaceted approaches available, supervised learning, unsupervised learning, and reinforcement learning stand out as pivotal techniques in navigating the complexities of financial analysis. They each maintain distinctive features and account for various financial data scenarios, making them essential tools in a CFA's toolkit.
Supervised Learning Techniques
Regression analysis
One of the cornerstones of supervised learning, regression analysis allows investors to model relationships between variables effectively. In finance, it’s commonly used to predict asset prices based on historical data. Its greatest strength is that it provides clear insights into how a dependent variable changes when any one of the independent variables is varied. For instance, a financial analyst might employ regression analysis to predict stock prices by considering various factors like earnings, interest rates, or economic indicators.
The key characteristic here is that regression analysis requires labeled input and output data, helping analysts identify trends and gauge the reliability of predictions. This makes it a popular choice, especially while conducting more formal evaluations of possible investment scenarios. However, it's vital to note that regression models assume a linear relationship, which might not always be the case in intricate financial landscapes. Thus, it can occasionally offer a skewed understanding if this assumption does not hold true.
Classification algorithms
Following closely behind, classification algorithms play a crucial role in decision-making processes within the CFA framework. These algorithms are designed to categorize data points into predefined classes based on their attributes. For instance, an analyst may classify loan applicants into 'high risk' or 'low risk' categories based on their credit history, income, and other factors.
The essential aspect that makes classification algorithms beneficial is their ability to handle complex datasets and deliver actionable insights. Unlike regression analysis, they do not require a continuous output, allowing them to work seamlessly with disparate data. Nevertheless, classification algorithms can also suffer from overfitting, where the model performs exceptionally well on training data but falls short on unseen data.
Unsupervised Learning Approaches
Clustering methods
Turning to unsupervised learning, clustering methods stand out as a powerful technique for identifying hidden patterns within datasets. These methods group similar types of data points together without any predefined labels, making them especially useful in exploratory data analysis within financial contexts.
The defining feature of clustering is its capability to reveal naturally occurring groupings in data. For instance, a financial analyst can use clustering to segment customer bases for targeted marketing strategies or risk assessment. On the downside, without labeled data, interpreting results can be more subjective than in supervised methods.
Dimensionality reduction
Another critical strategy within unsupervised learning is dimensionality reduction. This technique simplifies complex datasets by reducing the number of variables while retaining the informative aspects of the data. It’s particularly beneficial in finance, where multitudes of factors can cloud decision-making processes. By lessening the dimensional space, analysts can focus on significant variables that impact outcomes.
The unique feature of dimensionality reduction lies in its ability to shed unimportant data, making further analysis more efficient. However, it can sometimes lead to loss of critical information if not managed properly, thereby hindering certain analytical results.
Reinforcement Learning Applications
Lastly, reinforcement learning has emerged as a promising methodology in CFA. This approach simulates a dynamic environment where algorithms learn from actions and their outcomes. It culminates in optimizing financial strategies through trial-and-error methods.
In a finance application, consider an algorithm attempting to determine the best trading strategy. By evaluating the outcomes of its trades, the algorithm adjusts its strategies for future trades, enhancing its effectiveness over time. While the potential for high returns is enticing, the complexity and requirement for vast amounts of data can pose substantial hurdles in implementing this methodology effectively in actual trading scenarios.
"Integrating machine learning methodologies not only streamlines financial data analysis but also opens the door to innovative approaches that reshape decision-making frameworks."
Practical Applications in Finance
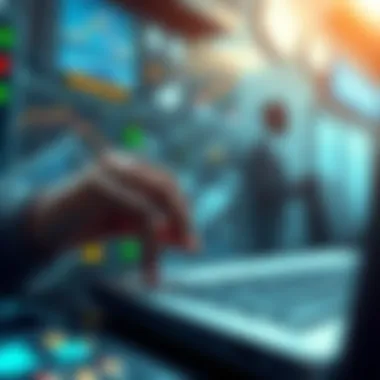
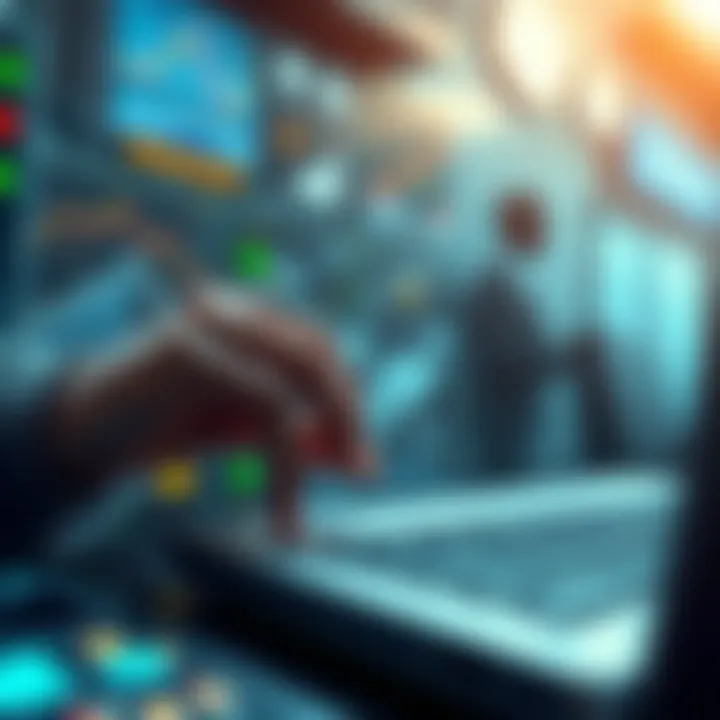
Integrating machine learning within the framework of Chartered Financial Analysis can significantly reshape the financial landscape. The practical applications of this fusion offer tangible benefits across various financial sectors, making it an indispensable topic for modern financial professionals. Let’s explore the nuanced insights into this significant integration by diving into three key applications: risk management strategies, portfolio optimization techniques, and algorithmic trading systems.
Risk Management Strategies
Risk management is pivotal in finance, and leveraging machine learning techniques can enhance these strategies remarkably. Traditionally, analysts rely on historical data and predefined risk models to assess potential losses and gain insights. However, the incorporation of machine learning enables a dynamic risk assessment approach.
Machine learning algorithms can analyze vast quantities of unstructured data, such as news articles, social media sentiments, and market trends, which were previously challenging to quantify. For instance, models that utilize natural language processing can gauge market sentiment, enabling analysts to predict and mitigate risks associated with stock volatility.
- Data-Driven Insights: Machine learning systems can furnish predictive analytics that enhances risk forecasting, allowing professionals to act proactively rather than reactively.
- Real-time Monitoring: Algorithms can continuously sift through new data, adjusting risk assessments on the fly. This agility can be vital during unpredictable market shifts.
However, professionals must remain vigilant about the quality of the input data. Garbage in, garbage out, as they say. Incorrect assumptions or poorly trained models can lead to devastating miscalculations in risk management.
Portfolio Optimization Techniques
A further application is in optimizing investment portfolios. Traditional portfolio management heavily relies on Markowitz’s mean-variance analysis. Machine learning introduces a more granular approach, where multidimensional data can be analyzed to discover patterns and correlations that may be overlooked in conventional frameworks.
For example, reinforcement learning can be employed to develop strategies that consider not only the expected returns but also the risk associated with various assets. Leaning on large datasets makes it possible to uncover previously unknown relationships among securities, refining the portfolio for higher returns and lower risks.
Some key benefits include:
- Enhanced Returns: Algorithms can facilitate asset allocation adjustments based on evolving market conditions, ultimately improving returns.
- Adaptive Strategies: The system learns from past decisions and outcomes, optimizing future choices based on real-world performance.
However, while machine learning can enhance portfolio performance, analysts must heed the potential for overfitting. If a model is too finely tailored to past data, it could yield poor results in future scenarios. A balanced approach to employing these techniques is vital for success.
Algorithmic Trading Systems
Lastly, let’s consider the boon that machine learning provides to algorithmic trading systems. This connection represents a confluence of technology and finance where customized algorithms execute trades on behalf of financial institutions, often in real time.
Machine learning enhances these systems by enabling them to learn from trading history. For instance, using supervised learning techniques, algorithms can be trained to recognize patterns in market movements that correlate with positive outcomes.
In terms of execution:
- High-frequency trading benefits from ultra-fast decision-making enabled by machine learning. Algorithms can exploit minuscule inefficiencies in the market much more efficiently than human traders.
- Strategies can dynamically adjust based on real-time data inputs, providing traders an edge over more static systems.
Yet, with great power comes great responsibility. The risks associated with algorithmic trading are substantial—market crashes can be exacerbated by poorly designed systems, resulting in significant financial fallout. The unpredictability of rapid trades and high market sensitivity necessitates utmost caution.
Challenges in Implementing Machine Learning in CFA
The integration of machine learning within the framework of Chartered Financial Analysis (CFA) is a fascinating venture, but it comes with its own share of hurdles. Understanding these challenges is crucial for analysts and investors who seek to leverage technology in their financial decisions.
Data Quality and Availability
One significant hurdle in this integration is ensuring the quality and availability of data. Machine learning thrives on vast amounts of data, and the performance of algorithms often hinges on the quality of input data. If the data is erroneous, biased, or incomplete, the models built upon it can lead to misleading conclusions. For financial analysts, the stakes are high. A model fed with low-quality data could suggest risky investments or flawed portfolio strategies.
Moreover, the world of finance is notorious for data silos, where valuable information is locked within proprietary systems. Finding accessible, high-quality datasets for training machine learning models can be challenging. Analysts might need to invest significant time and effort just to gather the right data—time that could otherwise be spent on actual analysis.
- Key considerations:
- Ensure data sources are reliable.
- Validate and clean the data before using it.
- Explore partnerships or subscriptions that provide enriched datasets.
Model Complexity and Interpretability
As the algorithms grow in complexity, so does the challenge of model interpretability. Financial analysts are often tasked with explaining their strategies to clients, and intricate models can become black boxes, offering little insight into their workings. This situation poses two-fold problems. First, if an analyst cannot decipher how a model arrived at a decision, it undermines trust in its conclusions. Second, regulatory bodies may demand explanations for investment choices driven by ostensibly opaque models.
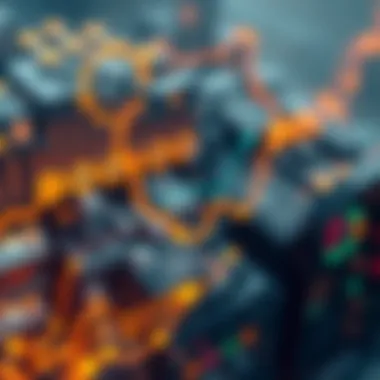
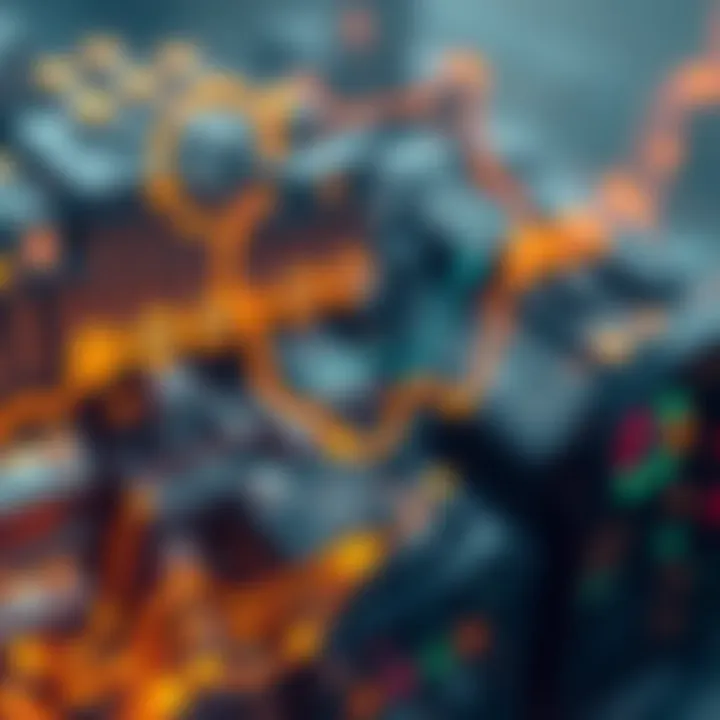
Finding a balance between sophisticated techniques and straightforward explanations is paramount. Some models might outperform simpler ones, but if analysts struggle to communicate their mechanics, they risk alienating stakeholders. Techniques such as Local Interpretable Model-agnostic Explanations (LIME) could be employed to better interpret complex models, but their adoption requires additional training and resources.
- Strategies to address complexity:
- Use simplified versions of complex models for initial analyses.
- Invest in training for stakeholders to understand advanced models.
- Leverage tools and methodologies aimed at improving interpretability.
Regulatory Constraints
Finally, regulatory constraints present another layer of complexity when integrating machine learning into CFA practices. The financial sector is heavily regulated, and any technological application must comply with a myriad of rules and standards. These regulations can vary significantly across different jurisdictions, making it a challenge for international firms to navigate.
Analysts must thoroughly understand the legal landscape and ensure that their machine learning practices do not run afoul of regulations that govern data privacy, algorithmic decision-making, and compliance reporting. The adoption of machine learning isn't just a technological challenge; it's also a regulatory maze. Ignoring these constraints can lead to reputational damage or legal repercussions.
- Considerations for compliance:
- Keep abreast of regulatory changes in various territories.
- Collaborate with legal experts when developing and applying machine learning techniques.
- Document all processes to ensure a clear audit trail is available.
In sum, while the challenges of implementing machine learning within CFA are numerous, they are not insurmountable. With careful planning and a strategic approach, analysts can effectively harness these technologies to enhance their analysis and decision-making capabilities.
Future Trends in CFA and Machine Learning
The landscape of finance is ever-changing, and as we look ahead, the relationship between Chartered Financial Analyst (CFA) principles and machine learning is poised to evolve at an unprecedented pace. Engaging with this topic is essential, as understanding these future trends can drive strategic decision-making for investors and analysts alike. The rapid advancements in technology are opening doors to new methodologies that enhance data analysis and investment strategies.
Emerging Technologies
Blockchain implications
Blockchain technology, characterized by its decentralized and secure nature, offers a robust framework for financial data integrity. It enables real-time tracking of transactions, ensuring transparency and reducing fraud. For CFA professionals, the importance of blockchain can't be overstated; it simplifies auditing processes and offers a more clear-cut view of the asset lifecycle. What sets blockchain apart is its immutability—once data is added, it cannot be altered without a peer consensus.
However, the implementation of blockchain has its challenges. The energy consumption for mining transactions is a concern, alongside the regulatory complexities in financial systems. Still, the benefits it brings, like enhanced security and operational efficiency, make it a popular choice in integrating with CFA practices. It pushes for a new wave of confidence in financial reporting that was simply unattainable through traditional methods.
Data analytics advancements
Advancements in data analytics are reshaping how CFAs assess and interpret financial information. The explosion of big data has made it imperative for finance professionals to harness powerful analytical tools to sift through vast amounts of information quickly. These tools provide insights that can lead to better decisions and strategies. The key characteristic of modern data analytics is its predictive power; it not only analyzes past performance but also forecasts future trends with remarkable accuracy.
One unique feature of these advancements is the ability to integrate structured and unstructured data sources. This capability allows for a richer analysis of market sentiments, consumer behaviors, and risk assessment. However, the race to become adept at using these data tools has its pitfalls. Complexity and reliance on technology may lead to inaccuracies if not managed carefully.
Evolving Financial Practices
In the face of these emerging technologies, financial practices must also evolve. CFAs need to embrace new tools and methodologies that integrate machine learning into their analysis. The emphasis will shift towards a more data-centric approach, pushing aside traditional metrics that have dominated for years.
The advent of machine learning in CFA not only optimizes existing practices but also lays groundwork for future innovations. As financial environments become progressively unpredictable, these evolving strategies will be crucial for maintaining a competitive edge.
Engaging with these trends isn’t just a necessity for efficiency—it’s a strategic imperative for those aiming to thrive in the financial sector.
End
The closing chapter of this exploration sheds light on how integrating Chartered Financial Analyst (CFA) principles with machine learning technologies stands as not just a trend, but a necessity in an increasingly digital financial landscape. As the finance industry continues to evolve, the capacity to leverage data-generated insights becomes paramount. Financial analysts and investors who can effectively wield these tools will likely find themselves at a significant advantage.
Recap of Key Insights
To summarize the key takeaways from our discussion:
- Significance of Integration: The fusion of CFA principles with machine learning not only enhances predictive accuracy but also empowers analysts to navigate complex market dynamics with greater confidence.
- Methodological Evolution: By adopting machine learning methodologies, CFA professionals can refine their investment strategies, making them more robust and adaptive to real-time data fluctuations.
- Practical Applications: From risk management strategies to portfolio optimization techniques, machine learning opens new avenues for efficiency and effectiveness in financial practices.
- Challenges Ahead: While the opportunities are vast, challenges related to data quality, model complexity, and regulatory constraints remain – aspects that must be continually addressed.
"Innovation is the ability to see change as an opportunity - not a threat."
– Anonymous
The Road Ahead for CFA Professionals
Looking towards the horizon, CFA professionals must prepare for a landscape transformed by technology. The future envisions:
- Continuous Learning: A commitment to lifelong learning will be critical for professionals aiming to stay current with unfolding technologies—whether it's grasping the nuances of advanced algorithms or understanding the ethical implications of automated decision-making.
- Collaborative Frameworks: Greater interdisciplinary cooperation, especially between financial experts and data scientists, will enhance innovation and lead to richer insights.
- Agility in Practice: With the pace of financial technology accelerating, being agile and adaptable will be imperative for addressing emerging challenges and seizing new opportunities.